Cyth Pairs AI Software with Robotic Arm to Sort Organic Seedlings
- markvasat
- Aug 2, 2022
- 3 min read
Updated: Oct 19, 2023

The Challenge
Agriculture is one of California’s largest industries. Due to the increasing costs associated with agriculture (land, water, labor), the industry is more frequently looking to automation as a potential solution for many of the processes of getting food from farm to table. One area in which traditional methods have begun to be replaced is handling, sorting, and processing raw plants. A client, representing multiple seedling nurseries within California, came to us with this exact need in mind. Utilizing Neural Vision software, we were able to use machine vision and deep learning to identify key plant features to optimize our client’s ability to determine plant viability.
The Solution
Integrating NeuralVision machine learning with a Denso SCARA pick-and-place robot we were able to automate the process by which seedlings are identified and selected for growth viability along an agricultural conveyor system.
The Story
Our client is a fruit seed starter and grower who nurtures seedlings to their midlife just before they bear fruit. While in this stage of development they are removed from the ground and guided via a conveyor line to sort which seedlings are considered of a high enough quality for distribution and planting at nurseries around the country. The identification process has traditionally been performed manually with workers stationed on an assembly line as they pick and choose plants that are healthy enough for packaging.
A decade ago, our client implemented an early attempt at artificial intelligence into their automated system to increase efficiency. They saw marginal success but were limited in their ability to handle the variation of plant breeds they encountered. Limited to a small image data set size, their original system was restricted in its capabilities and lacked customization options. The customer was looking for cutting-edge technology that would give them greater control, trainability, and handling when paired with a pick-and-place robot for seedling processing. Recognizing Cyth’s expertise and track record of integrated solutions they approached us with their needs in mind.
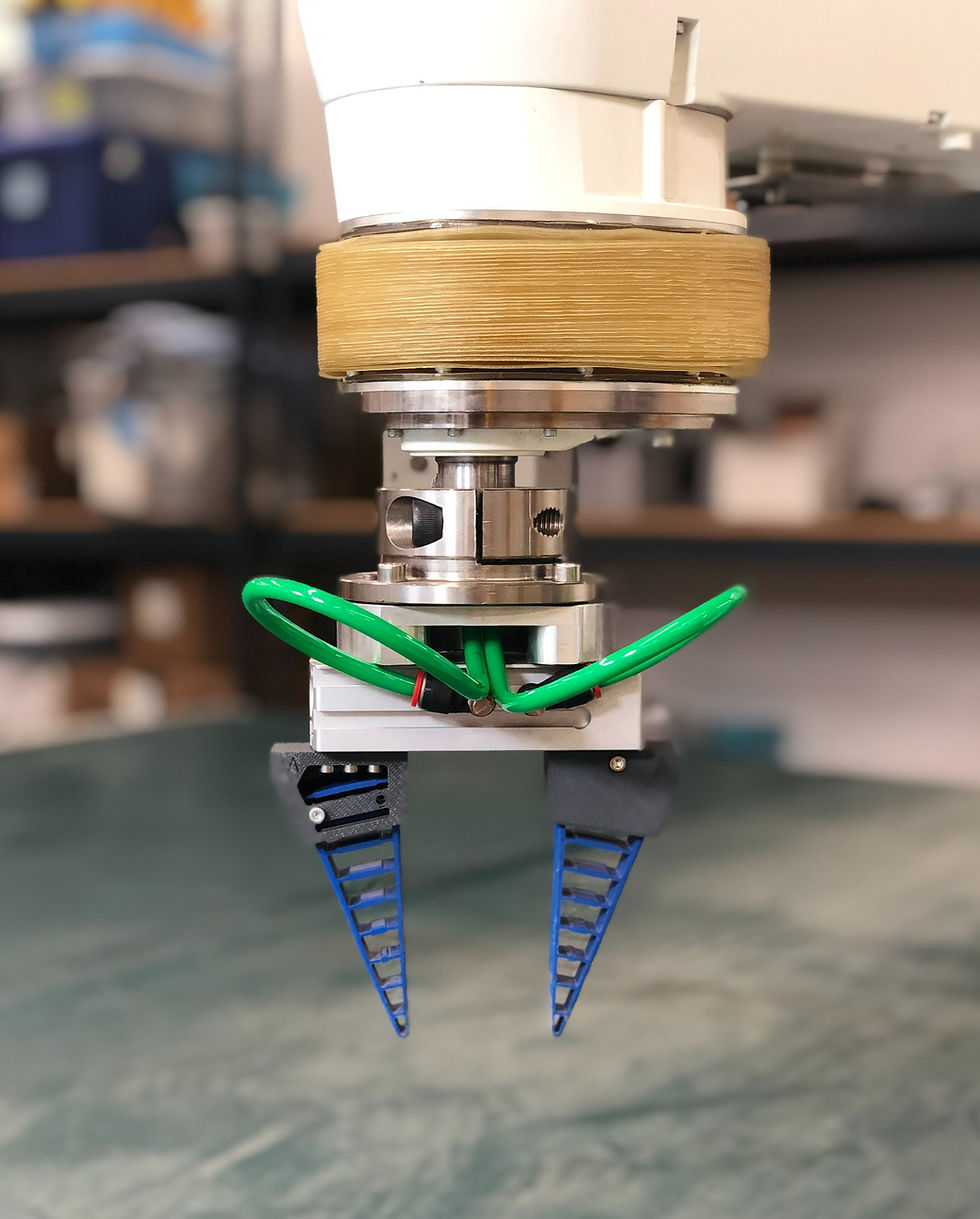
The Cyth Process
The project started with an initial prototype – Phase Zero, capturing images of raw seedlings for testing and evaluation in Neural Vision. Leveraging Deep Learning, Neural Vision builds a model pixel by pixel to sort, grade, and classify objects. This way, the client has total control of their system’s performance without having to depend on software engineers to adjust or modify the code. The software was designed to allow a person with no experience to be able to train their system to inspect and classify products with a simple click.

Upon Phase Zero’s success, our engineering team moved to the next phase where we designed a vision robot assembly to work along the client’s conveyor. Our engineers began the mockup by pairing a Denso SCARA robot arm with the conveyer. After optimizing lighting, cameras, and lenses our team began to run test samples to see the vision system’s performance and the accuracy with which the claw was able to select seedlings.
Overcoming the Obstacles
The greatest challenge our engineers faced along the project timeline was pairing our Neural Vision with the high-accuracy conveyor tracking. Pairing a machine vision system with a conveyer presents difficulties as the robot arm has to take into account the displacement distance of the moving belt. This required calibrating the robot arm to the vision system as well as calibrating it to the moving belt using algorithms in the software. We collaborated with Denso’s robotics team to learn how to best utilize the robot's features for a fully integrated solution of our hardware, software, and their robot. After fine-tuning the Denso SCARA robot and Neural Vision’s capabilities, the system worked seamlessly to identify, pick, and place off of the conveyer the seedlings that met the client’s criteria.
Delivering the Outcome
By partnering with integration firms like Cyth farms can apply modern integration techniques and processes to more efficiently deliver food from farm to table. The client has been thrilled with the integration of robots and Neural Vision machine learning into their processing line as they have successfully scaled it into multiple conveyors. From identification to categorization and even decision making, Neural Vision and the Cyth Solution has given our clients and the agricultural industry alike a new step forward in the automation of their plant processing systems.
Technical Specifications
· Cyth’s Neural Vision Software
· LabVIEW 2017 32-bit
· 2 x Basler 5MP, 17fps, Area Scan, CCD Global Shutter, Color Camera
· 2 x Edmund Optics 16mm, 300-2000mm Primary WD, HP Series Fixed Focal Length Lens
· 1 x Medium Size 4-Axis SCARA HM-G Series Denso Robot
· 1 x Incremental, Resolution 1000P/R, 40-dia., 5 to 5 VDC, Line driver output, Pre-wired models (2 m) OMRON Encoder
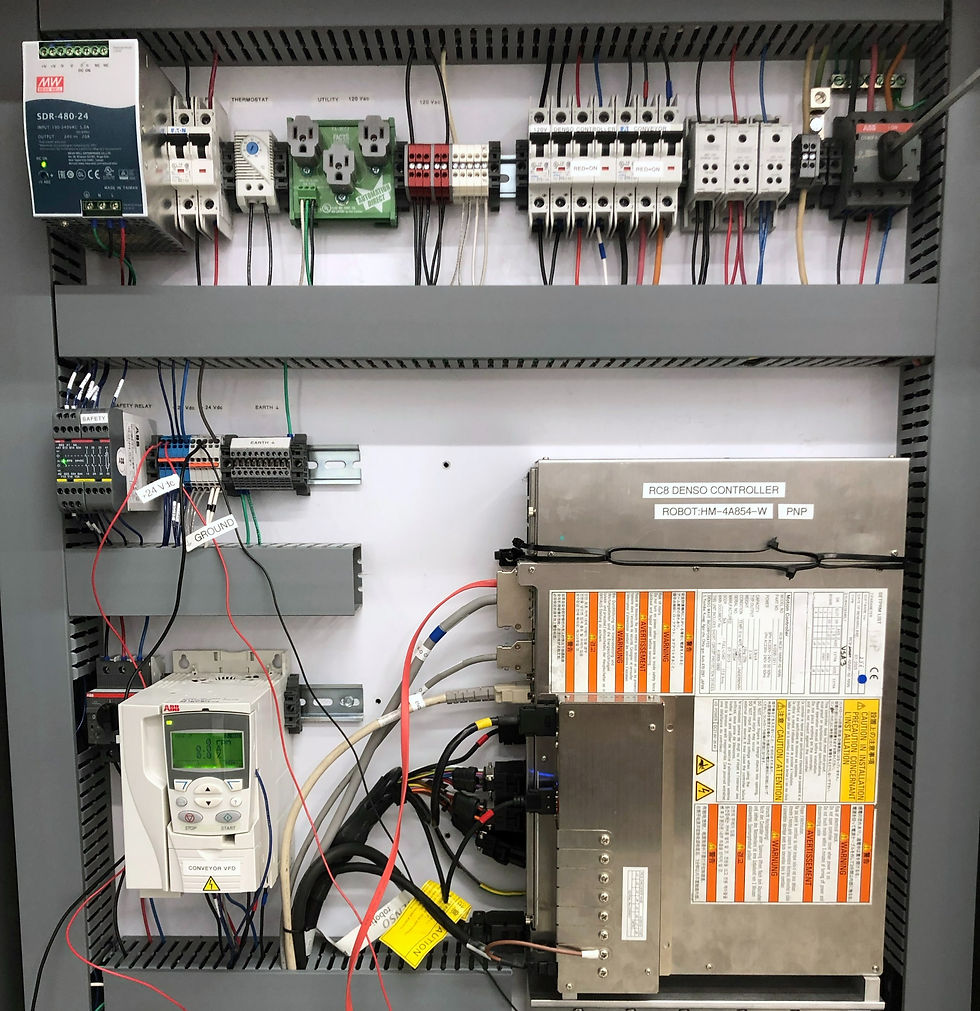
Comments